Data analytics is evolving rapidly, driven by advancements in real-time processing, predictive models, and AI. The future in data analytics will see businesses leverage these technologies for more efficient decision-making and competitive advantages. This article delves into the upcoming trends shaping the field and examines their applications across different sectors.
Top Trends Shaping the Future in Data Analytics
Data analytics is undergoing a transformation driven by advancements in real-time processing, predictive models, and AI technologies. These innovations are redefining how businesses make decisions and gain competitive advantages. In this article, explore the emerging trends in data analytics and their applications across various sectors.
Key Takeaways
- Real-time data processing provides immediate insights, enhancing operational efficiency and enabling faster decision-making across industries.
- Predictive analytics, integrated with AI and machine learning, allows organizations to forecast outcomes, optimize operations, and refine strategies.
- Data democratization through augmented analytics and no-code solutions empowers non-technical users to independently engage with data, promoting a data-driven decision-making culture.
Real-Time Data Processing: The New Norm

Real-time data processing has become a pivotal development in data analytics. It allows companies to analyze data as it is generated, providing instant insights that significantly boost operational efficiency. This capability offers businesses a competitive edge by enabling quick responses to evolving customer behaviors and market trends.
The integration of real-time data into business operations, known as continuous intelligence, is transformative in sectors like logistics, finance, and healthcare. Real-time analytics empowers businesses to make data-driven decisions that are both smarter and more timely, thus staying ahead of competitors.
Applications in Finance and Social Media
In finance, real-time data processing enhances fraud detection by immediately identifying suspicious activities, thereby safeguarding both organizations and customers.
Social media platforms use real-time analytics to optimize content delivery and increase user engagement. Edge analytics processes data near its source, boosting operational efficiency for these platforms.
Benefits for Supply Chain Management
Real-time data processing is revolutionizing supply chain management by reducing latency and improving logistics. Companies like Walmart and UPS utilize real-time analytics to streamline operations and better meet customer demands.
UPS, for instance, adjusts delivery routes based on live data inputs such as package tracking, traffic, and weather conditions, ensuring timely deliveries and cost reductions.
Predictive Analytics: Anticipating Future Trends
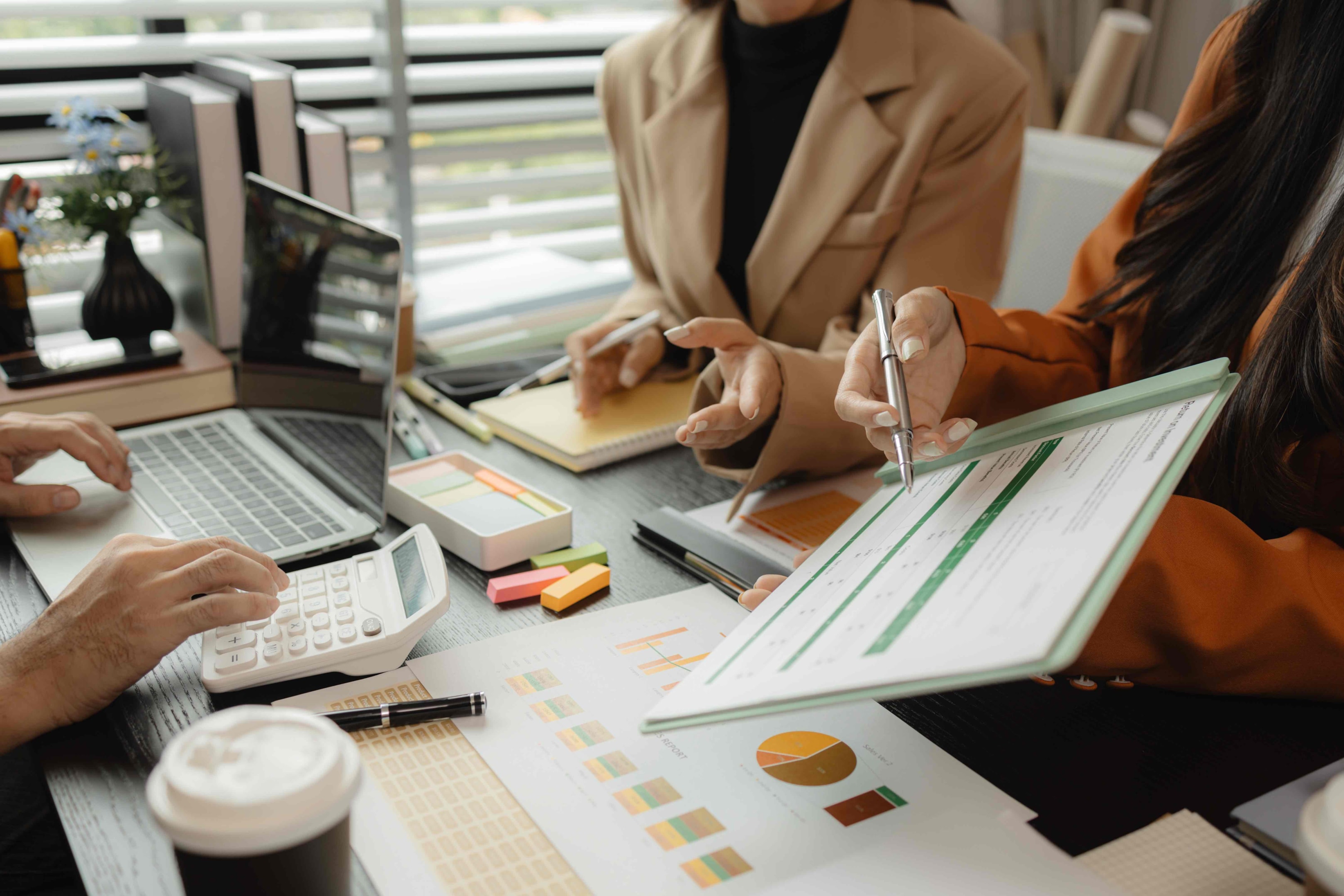
Predictive analytics leverages historical data to forecast near-term outcomes, enabling businesses to make informed decisions. This powerful tool helps optimize operations, reduce risks, and increase profits. By merging predictive with prescriptive analytics, companies can anticipate trends, navigate uncertainties, and capitalize on opportunities.
The integration of AI and machine learning into predictive analytics is increasing, enhancing analytical capabilities. Over 70% of businesses using predictive analytics report improved forecasting accuracy, leading to better management decisions and strategic planning.
Predictive analytics is poised to play a crucial role in the future of business and technology.
Use Case: Retail Industry
In retail, predictive analytics enables businesses to forecast customer demand by analyzing historical data. Understanding customer behavior and preferences allows retailers to personalize marketing campaigns and customer experiences, driving engagement and sales.
Impact on Healthcare
Healthcare providers are adopting predictive analytics to improve patient outcomes and optimize resource allocation. For example, Massachusetts General Hospital uses predictive analytics to identify high-risk patients and enhance intervention programs, reducing readmissions by 22%.
Similarly, Kaiser Permanente employs predictive analytics to improve healthcare outcomes by lowering patient readmissions.
Augmented Analytics: Empowering Non-Technical Users

Augmented analytics democratizes data insights by making advanced analytics accessible to non-experts through AI and machine learning. This approach uses AI, machine learning, and natural language processing to enhance data analyses and decision-making. Non-technical users can conduct complex analyses without deep technical knowledge, transforming the data analytics landscape.
Adopting augmented analytics accelerates data-driven insights and enhances accessibility across organizations. Teams can extract insights without specialists, streamlining decision-making and improving operational efficiency.
Examples of Augmented Analytics Tools
Leading augmented analytics platforms like Tableau, Microsoft Power BI, and IBM Cognos Analytics make data analysis more accessible. These tools harness AI and machine learning to streamline data analysis, enabling users to generate insights quickly and efficiently through advanced data analytics and visualization.
This approach fosters a culture of data-driven decision-making, empowering non-technical users to independently engage with data.
Enhancing Strategic Decision-Making
Implementing augmented analytics tools leads to quicker insights, enhancing decision-making capabilities across organizations. This improves operational efficiency, drives innovation, and increases agility, providing a significant competitive advantage.
Nearly half of businesses are using AI-powered data analytics platforms to boost strategic decision-making processes.
Ethical Considerations in Data Analytics
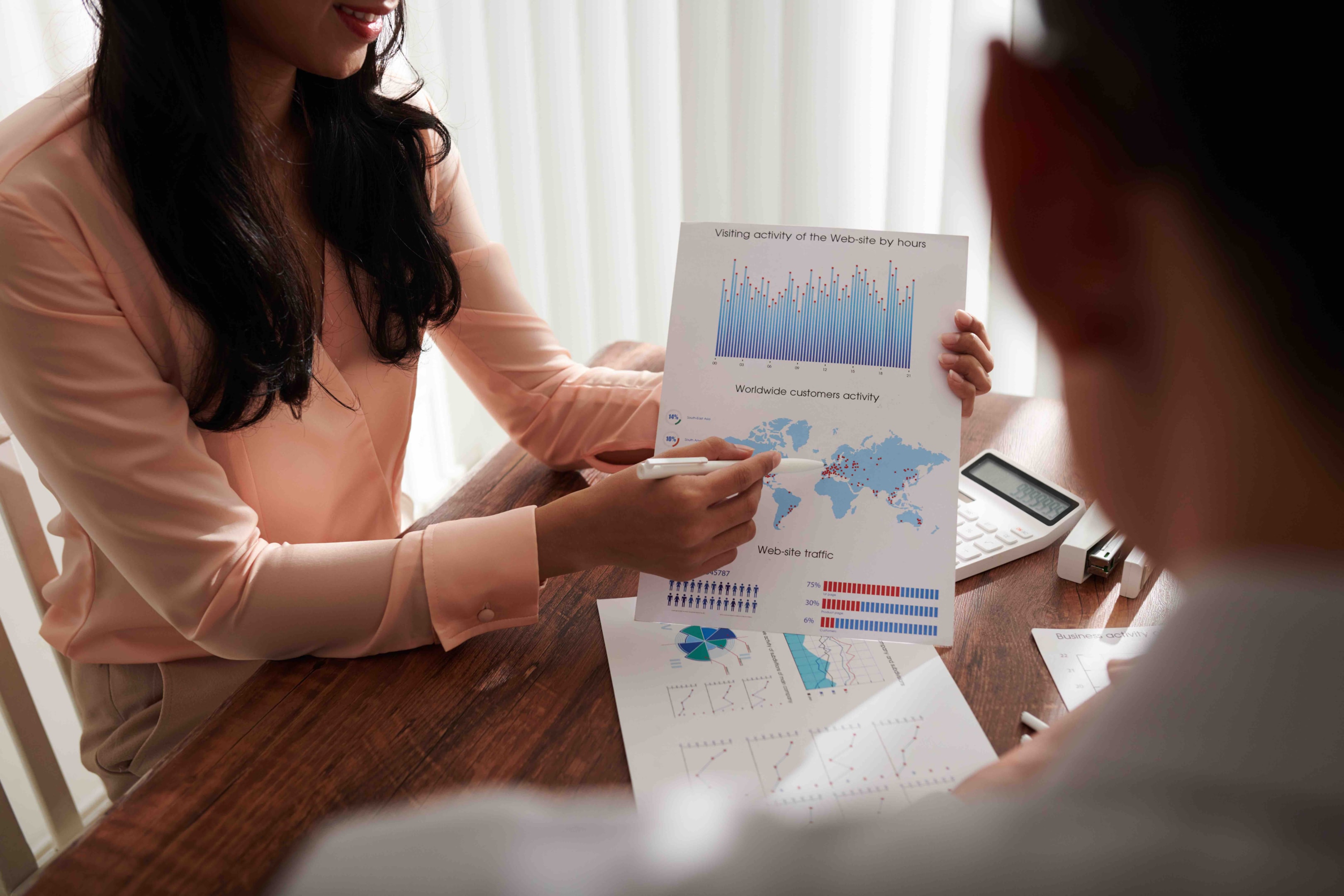
The growing reliance on data analytics brings critical ethical challenges, including privacy protection, data accuracy, and security maintenance. Regulations like GDPR and CCPA require organizations to prioritize data privacy and governance to avoid legal penalties and enhance credibility. Data analysts should emphasize data security and compliance with these regulations due to increasing privacy concerns.
Businesses must examine their data pipelines to ensure data health and compliance. Ethical considerations in data analytics are crucial for maintaining trust and integrity in business.
Local processing of customer data helps maintain security and privacy by minimizing exposure during transmission.
Importance of Data Governance
Robust data governance frameworks are vital for ensuring compliance with privacy regulations and maintaining data integrity. Effective governance helps organizations avoid legal penalties and boosts market credibility. Compliance with regulations like GDPR and CCPA protects company reputation and ensures data security.
Insurers are implementing strong governance frameworks and explainable AI models to enhance data governance.
Ethical AI Practices
Ethical AI practices ensure fair and unbiased decision-making processes, enhancing trust in AI systems. Future data analysts must navigate privacy laws, ethical usage, and security to ensure responsible AI practices.
Organizations focus on DEI (Diversity, Equity, and Inclusion) analytics to foster inclusive work environments, promoting fair decision-making. As insurers increasingly rely on AI, transparency, unbiased systems, and accountability are emphasized.
Data Democratization: Breaking Down Barriers

Data democratization breaks down barriers, granting broader access to data and analytics tools across organizations. This trend empowers non-technical audiences to independently engage with data, fostering a data-driven decision-making culture. Organizations invest in training and resources to enhance data literacy, recognizing that a data-literate workforce can effectively leverage data.
A structured framework for data literacy training enables organizations to maximize their data capabilities. The future of predictive analytics will involve more sophisticated tools that democratize access for businesses of all sizes, making advanced analytics more accessible and impactful.
Role of No-Code Solutions
No-code solutions play a pivotal role in democratizing data insights by removing the need for coding knowledge in data processes. These platforms allow users to interact with data tools through natural language queries, making data analysis more accessible.
No-code and low-code tools empower stakeholders to independently engage with data, transforming data utilization within organizations.
Impact on Business Culture
Data democratization fosters a collaborative environment by allowing employees at all levels to utilize data for informed decision-making. No-code solutions facilitate collaboration by enabling employees without technical expertise to analyze data and share insights effectively.
Training programs focused on data literacy enhance the workforce’s ability to make data-informed decisions, promoting engagement and performance. These elements drive innovation, provide a competitive advantage, and reshape business culture.
Advanced Analytics Tools and Techniques
Integrating AI and machine learning transforms data analysis, automating model building and eliminating manual intervention. These technologies offer deeper insights, trend prediction, and action prescription, enhancing forecasting accuracy. Emerging trends like Generative AI and retrieval-augmented generation are pushing the boundaries of big data analytics.
Generative AI creates synthetic datasets and automates content creation, while retrieval-augmented generation augments AI models with real-time data for accurate insights.
In 2024, technologies driving data analytics include AI, machine learning, NLP, data mesh, edge computing, and cloud technologies.
Integration of AI and Machine Learning
AI and machine learning revolutionize data analytics by transforming data interpretation and utilization within organizations. These advanced data science tools provide deeper insights and enhance forecasting accuracy, enabling businesses to make data-driven decisions with greater confidence.
Automating model building and eliminating manual intervention make data analysis more efficient and effective.
Quantum Computing in Data Analytics
Quantum computing uses quantum mechanics to process information differently than classical computing, offering revolutionary advancements in data analytics. Its ability to handle and analyze complex, large datasets at unprecedented speeds provides significant advantages.
Sectors like finance, healthcare, and logistics benefit from quantum computing’s rapid data processing capabilities, allowing for insights previously unattainable. As quantum technology matures, its integration into data analytics will transform decision-making processes and provide a competitive edge across industries.
Edge Analytics: Processing Data at the Source
Edge analytics processes data near its source, allowing for quicker insights and reduced congestion in centralized systems. This approach benefits IoT applications by reducing latency and bandwidth usage, crucial for real-time decision-making. Processing data closer to the source, edge analytics minimizes travel time, significantly enhancing operational efficiency and response times.
Industries like manufacturing and healthcare, which have successfully implemented edge analytics, report significant improvements in operational efficiency and responsiveness to real-time data. This trend is essential for businesses looking to enhance their competitive advantage and operational efficiency.
Benefits for IoT Applications
Edge analytics enhances IoT applications by reducing latency, decreasing bandwidth usage, and improving response times. By processing data closer to its source, edge analytics minimizes the time it takes for data to travel, thereby significantly reducing latency. This capacity to analyze data at the edge enhances response times, allowing for quicker decision-making in IoT applications.
For manufacturers, implementing edge computing reduces latency and improves real-time decision-making processes.
Real-World Use Cases
Edge analytics significantly impacts various industries, including manufacturing, retail, and smart cities. In manufacturing, edge analytics enables real-time monitoring of equipment performance, preventing failures and optimizing maintenance schedules. In retail, it allows for personalized customer experiences by analyzing shopping behaviors on-site.
Smart cities use edge analytics to manage traffic flow and optimize public transportation systems, showcasing its broad applicability. Adopting edge analytics is essential for businesses seeking to enhance operational efficiency and gain a competitive advantage.
Data Literacy: A Crucial Skill for the Future
With accelerating data creation, the ability to interpret and utilize data effectively has become paramount for making informed decisions. Data literacy empowers individuals across organizations to engage with data, fostering a data-driven decision-making culture. Critical thinking and problem-solving skills are essential for analyzing data, ensuring valid interpretation, and deriving meaningful insights. Data analysts play a crucial role in shaping business strategies by identifying market trends, customer behaviors, and operational efficiencies.
Organizations are investing more in training and resources to enhance data literacy among employees. A structured framework for data literacy training, including workshops, online courses, and mentorship programs, significantly improves an organization’s capability to leverage data effectively.
Understanding data governance is crucial for future data analysts to ensure privacy laws and ethical usage are maintained.
Training Programs and Initiatives
Organizations prioritize training programs to enhance data literacy among employees, recognizing the value of a data-literate workforce. Training initiatives, including workshops, online courses, and mentorship programs, provide employees with the skills needed to analyze data effectively.
Domain knowledge helps data analysts perform better as understanding what to analyze improves job performance.
Role in Enhancing Business Performance
Data literacy is essential in the modern business environment as organizations increasingly rely on data-driven decision-making. A workforce equipped with data literacy skills enhances business outcomes by leveraging insights for better strategy formulation, providing a competitive edge.
Investing in data literacy training boosts individual employee capabilities and fosters a culture of informed decision-making and innovation, leading to significant competitive advantages.
Summary
The future of data analytics is being shaped by transformative trends, including real-time data processing, predictive analytics, augmented analytics, and ethical considerations. Real-time data processing offers immediate insights, enhancing operational efficiency and competitive advantage. Predictive analytics helps companies anticipate future trends and make informed decisions, while augmented analytics democratizes access to advanced data insights for non-technical users.
Ethical considerations and data governance are critical for maintaining data integrity and regulatory compliance. Data democratization and no-code solutions foster collaboration and innovation. Advanced analytics tools and techniques, such as AI, machine learning, and quantum computing, revolutionize data processing and analysis. Edge analytics enhances IoT applications by reducing latency and improving real-time decision-making. Finally, data literacy is crucial for the future, enabling organizations to leverage data effectively and gain a competitive advantage. The journey into the future of data analytics is both exciting and full of potential, promising to transform how businesses operate and make decisions.
Frequently Asked Questions
What is real-time data processing, and why is it important?
Real-time data processing analyzes data as it is generated, enabling businesses to gain immediate insights and respond swiftly to changes. This capability is crucial for enhancing operational efficiency and maintaining a competitive edge in today's fast-paced environment.
How does predictive analytics benefit the retail industry?
Predictive analytics benefits the retail industry by enabling retailers to forecast customer demand through historical data analysis, allowing for tailored marketing campaigns and personalized customer experiences that drive increased engagement and sales.
What are some popular augmented analytics tools?
Popular augmented analytics tools include Tableau, Microsoft Power BI, and IBM Cognos Analytics, which utilize AI and machine learning to simplify data analysis for non-technical users.
Why is data governance important in data analytics?
Data governance is crucial in data analytics as it ensures compliance with privacy regulations and maintains data integrity, safeguarding organizations from legal penalties and bolstering their market credibility.
How does edge analytics benefit IoT applications?
Edge analytics significantly enhances IoT applications by enabling quicker decision-making through reduced latency and decreased bandwidth usage, leading to improved response times and more efficient data handling.