Business Intelligence (BI) and Big Data together can transform your decision-making process. This article will show you how to combine their strengths to optimize performance and stay competitive.
Top Techniques for Leveraging BI and Big Data for Better Business Decisions
Business Intelligence (BI) and Big Data together can transform your decision-making process. This article will show you how to combine their strengths to optimize performance and stay competitive.
Key Takeaways
- Integrating Business Intelligence (BI) with Big Data analytics allows organizations to transform raw data into actionable insights, enhancing decision-making and driving growth.
- Employing predictive analytics powered by Big Data enables businesses to forecast trends and prepare for future challenges, improving operational efficiency.
- Implementing best practices in data management and visualization optimizes the performance of BI tools and enhances user engagement with data-driven decision-making.
Understanding Business Intelligence and Big Data

Business Intelligence (BI) processes structured data from internal sources, emphasizing efficient reporting and analysis through tools like data visualization and data warehousing. Over the years, BI has evolved from static reports to more interactive and self-service analytics, allowing for faster data-driven decisions. This transformation has made it easier for business users to interact with data and derive insights without deep technical knowledge.
Big Data, on the other hand, is defined by its volume, variety, and velocity, encompassing both structured and unstructured data such as text, images, and sensor readings. This diversity allows businesses to gather a more comprehensive view of their operations and customer behaviors. While BI systems aggregate historical data to provide performance insights, Big Data analytics processes real-time information, enhancing operational responsiveness.
Integrating BI with Big Data analytics enables organizations to transform raw data into actionable insights, leading to improved decision-making and strategic innovation. This synergy allows businesses to adapt their strategies based on current market conditions and customer needs, driving growth and innovation.
Leveraging both BI and Big Data, organizations can enhance decision-making, optimize operations, and stay competitive in the evolving market landscape.
The Role of Big Data in Enhancing BI
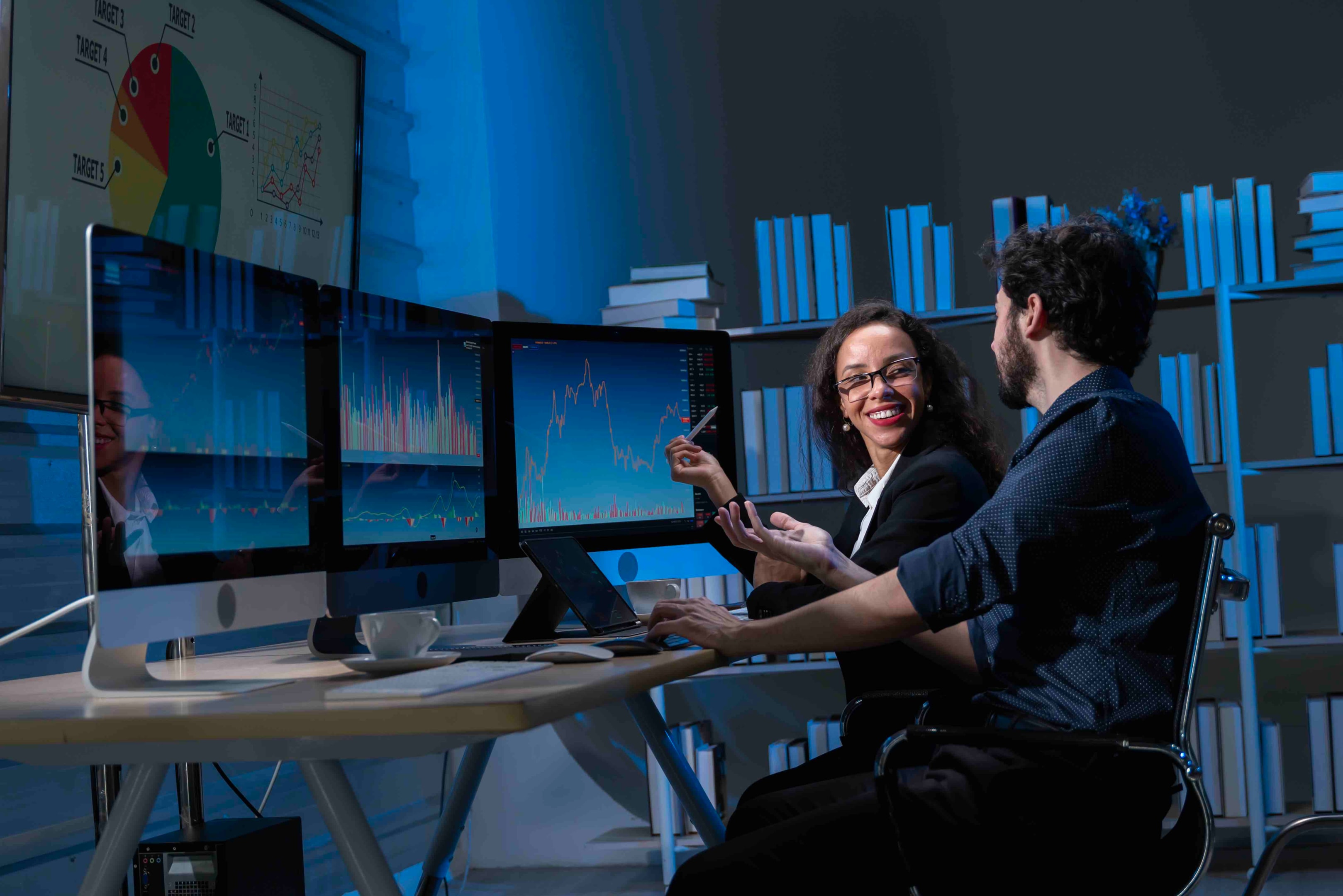
Big Data analytics enhances business intelligence by deriving actionable insights from structured and unstructured data, leading to better decision-making and deeper operational understanding. For instance, analyzing larger datasets helps businesses uncover hidden patterns and optimize their operations.
Integrating Big Data into BI strategies offers insights into customer needs and behaviors, enhancing personalization and predicting preferences more effectively. This integration is paramount for companies looking to innovate and stay ahead in a competitive market.
How Big Data Fuels BI Strategies
Big Data provides the raw material for informed decisions and enhances operational efficiency. Small businesses that leverage Big Data experience improved customer experiences, better operational outcomes, and increased support for data-driven decision-making. The real-time data generated by IoT devices can be used for advanced analysis in BI strategies.
Analysts employ various techniques, such as classification, clustering, regression, and association rule learning, for effective data mining of customer data. Through data collection, they can uncover customer preferences, purchasing habits, and pain points.
Effective data visualization tools simplify complex data, aiding faster decision-making and improving business strategies.
Predictive Analytics with Big Data
Predictive analytics forecasts future trends and market shifts using historical data patterns. Machine learning enhances data analysis by enabling predictive modeling that identifies trends from historical data. Analysts use algorithms in predictive analytics to forecast market trends, demand, and risks.
Analyzing historical data with predictive analytics identifies patterns and forecasts market trends, enabling informed decisions about future conditions. This approach not only drives growth but also improves efficiency by enabling organizations to prepare for future challenges and opportunities.
Key Technologies in BI and Big Data

The main technologies supporting Big Data and Business Intelligence include data warehousing, machine learning, and advanced analytics. The integration of Big Data analytics with technologies like AI and IoT enables businesses to automate decision-making and improve efficiency.
BI platforms utilize advanced analytics, data mining, and business intelligence tools to analyze data, including the use of a bi tool. For example, Microsoft Power BI is equipped with robust capabilities to handle and analyze large datasets.
Advanced data visualization tools and data tools enhance user interactions with data through interactive features and diverse visual elements, making it easier to derive valuable insights and make data-driven decisions.
Data Warehousing for Big Data Storage
Data warehouses serve as centralized repositories for processed data, facilitating efficient analysis and reporting. Data warehousing is an essential practice for storing and managing large datasets within business intelligence frameworks. This centralization improves data accessibility, enabling businesses to leverage insights from their data more effectively.
Data warehouses improve the ability to analyze and report on large datasets, enabling better data-driven decisions. Data warehouses provide a structured environment for data storage, supporting robust analysis and reporting for effective BI strategies.
Machine Learning in Data Analysis
Machine learning algorithms are adept at spotting patterns, making predictions, and learning from new data, thereby improving overall data processing capabilities. This technology significantly enhances the analysis of complex data, enabling organizations to derive deeper insights that can inform business decisions.
Leveraging machine learning, businesses can uncover hidden trends in datasets not visible through traditional analysis. These insights lead to informed decisions and better strategic planning, driving business growth and innovation.
Best Practices for Managing Large Datasets in BI
Implementing performance filters early in dataset queries can significantly enhance performance. Optimizing the data refresh process is crucial for managing large datasets in BI effectively. Key aspects of managing Big Data in Power BI involve handling data and optimizing report/dashboard performance. Ethical data governance focuses on ensuring data quality and security while addressing privacy and ethical concerns in data handling.
Data storytelling combines narrative techniques with data visualization to provide context and insights that lead to actionable strategies. Self-service analytics empowers users to perform data analyses and generate reports independently, reducing reliance on IT teams.
Data Modeling Techniques
Efficient data modeling plays a crucial role when dealing with Big Data in Power BI. It is essential for optimal performance and analysis. Data modeling in Power BI should aim for accurate data representation and performance optimization. By minimizing data loading and selecting only necessary columns, analysts can improve data processing efficiency.
Data mining techniques help analysts identify hidden patterns in customer data to inform business strategies. Effective data visualization tools streamline complex data interpretation, analyzing data to support quicker decision-making and enhancing overall business strategies.
Using Aggregations for Performance Optimization
Aggregations condense detailed data into summary tables, which effectively reduces the volume of data that needs to be processed. By summarizing data, aggregations directly enhance the speed and efficiency of BI tools when analyzing large datasets.
Power BI includes tools like Performance Analyzer and DAX Studio. These tools assist in identifying and addressing performance bottlenecks. These tools analyze query times. They also optimize DAX expressions to improve overall performance. Utilizing aggregations in conjunction with performance tuning tools significantly improves the performance of business intelligence applications.
Real-Time Data Processing in BI
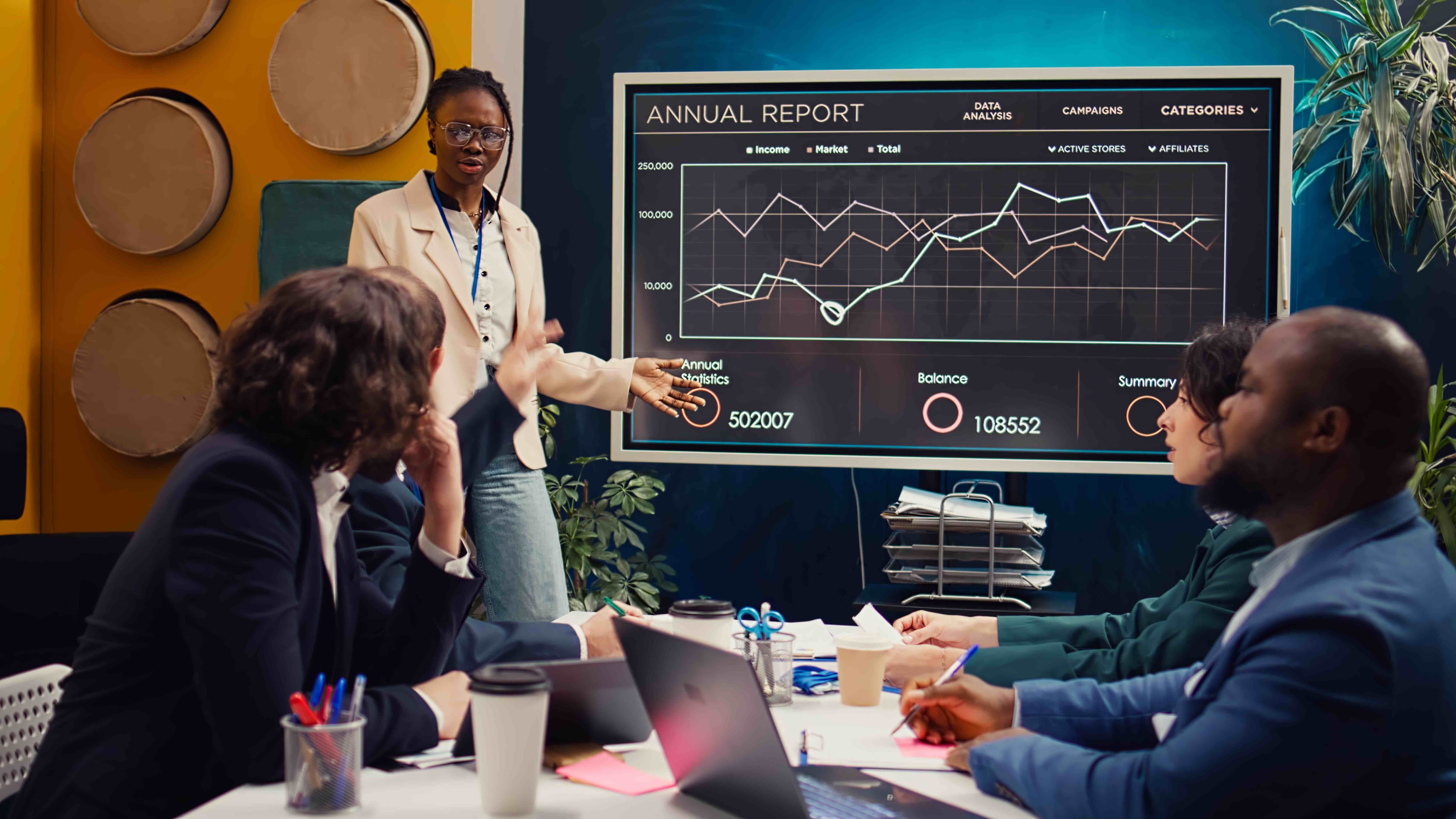
Real-time data processing is a key component of modern business intelligence, enabling organizations to harness the power of immediate insights from their data. Real-time analytics helps businesses make quick, informed decisions based on the latest data, enhancing their responsiveness and agility.
Real-time analytics helps businesses immediately identify and rectify operational inefficiencies, boosting effectiveness and efficiency. For instance, the use of real-time analytics can optimize logistics by allowing businesses to adjust their supply chain operations dynamically to meet current demands.
Case Studies: Companies Leveraging BI and Big Data
Big Data analytics and business intelligence are essential components for remaining competitive in the digital age. Companies that integrate Big Data analytics with BI gain a competitive advantage. For example, IBM has seen improved operational efficiencies, product innovation, and a competitive stance through its Big Data solutions.
Netflix develops a sophisticated BI platform to understand user preferences and enhance customer satisfaction.
Charles Schwab utilizes business intelligence to gain a comprehensive view of all branches and identify performance metrics.
Starbucks uses Big Data and BI tools to optimize store locations and enhance customer experiences.
Amazon's Use of Big Data for Personalized Recommendations
Amazon utilizes Big Data analytics to analyze customers’ purchase history, search patterns, and shopping cart contents for personalized recommendations. These personalized recommendations drive sales and enhance customer engagement on Amazon’s platform.
The data points that Amazon analyzes for its recommendations include individual purchase histories and customer behavior patterns. Personalized recommendations are crucial for enhancing customer experience in e-commerce.
UPS's Route Optimization with Big Data
UPS employs analytics to streamline delivery processes and manage its vehicle fleet effectively in its business operations. Optimizing delivery routes, UPS enhances customer service with timely deliveries.
UPS is a leading logistics company that leverages Big Data analytics to streamline its operations. These optimizations improve operational efficiency, enhance customer service, and reduce costs.
Future Trends in BI and Big Data

Emerging trends in BI and Big Data include AI-driven analytics, real-time data streaming, IoT, and edge computing. Artificial intelligence and machine learning are expected to continue growing in business intelligence applications.
Data visualization tools are essential for collaboration across teams and departments in BI. These emerging trends will significantly influence future business decisions by enhancing data analysis and collaboration capabilities.
Building a Data-Driven Culture
Leadership commitment is essential for fostering a data-driven culture, setting the standard for data practices within the organization. Organizations should prioritize enhancing employees’ data literacy through training, enabling them to effectively interpret and utilize data.
A data-driven culture depends on the availability and sharing of data across stakeholders to facilitate decision-making. Collaboration among teams on data initiatives enhances communication and leads to better decision-making and outcomes.
Summary
Leveraging BI and Big Data offers unparalleled opportunities for businesses to make informed decisions and drive innovation. Key techniques include the integration of advanced technologies like machine learning and data warehousing, as well as best practices for managing large datasets. Real-world examples from companies like Amazon and UPS illustrate the transformative power of these tools.
By adopting these strategies, businesses can enhance operational efficiency, personalize customer experiences, and stay ahead in a competitive market. The future holds even more promise with emerging trends like AI-driven analytics and real-time data streaming. Embrace the data-driven revolution and lead your organization to new heights.
Frequently Asked Questions
What is the difference between Business Intelligence and Big Data?
The key difference is that Business Intelligence focuses on structured data for reporting and analysis, whereas Big Data encompasses both structured and unstructured data, enabling real-time insights.
How does predictive analytics benefit businesses?
Predictive analytics empowers businesses to make informed decisions by analyzing historical data and forecasting future trends, ultimately enhancing strategic planning and operational efficiency.
What role does data warehousing play in BI?
Data warehousing serves as a centralized repository that enhances data accessibility, facilitating efficient analysis and reporting in business intelligence (BI). This structure ultimately supports informed decision-making.
How do companies like Amazon use Big Data?
Amazon leverages Big Data to analyze customer behavior, delivering personalized recommendations that boost engagement and sales. This approach fosters a tailored shopping experience that keeps customers returning.
What are some emerging trends in BI and Big Data?
Emerging trends in BI and Big Data prominently feature AI-driven analytics, real-time data streaming, Internet of Things (IoT), and edge computing, all of which significantly enhance data analysis and decision-making capabilities.